Data Annotation And Machine Learning
About the Client
Client was an aggregator for sourcing mined data for the businesses in the area of entertainment, autonomous vehicles and multi-lingual translation services. We helped them with a single platform driven solutions approach, with managed services, as a single stop solution shop.
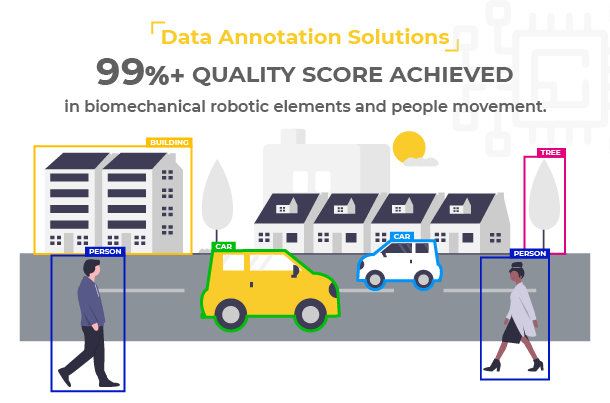
Challenges
- The process must track a person’s every small movement, robotic elements. And do a bio-mechanical analysis in real time.
- The process must simply identify which objects and other properties exist in an image, find the position of individual objects and recognize and understand what's in the image at the pixel level.
- With higher volumes to be delivered in a short duration of time with 95% accuracy was a challenge.
- Estimated 50 people were required to be hired and trained in a week’s time.
Solution
- Explored platforms and best fit solution for the varied needs.
- A single platform with 80% ML led annotation, for 2D/3D bounding boxes and segmentation was proposed.
- For varied skillset needs, multi-site operations executed for data annotation and labelling purpose. This also helped in quick hiring, with remote training options.
- More than 3 different programs integrated in single platform, with easy end-to-end API integrations.
Result
- Implementation and delivery done within defined timeline
- Handled and processed approx. 100k+ images.
- Quality achieved beyond the client’s benchmark of 95% to 99.00% with 100% quality checks.
FiveS Digital is an impact sourcing BPM partner for it’s global clients, helping them improve their CX every single time. Thus, generating on-ground impact by creating job opportunities where people belong.
How we did it?
Benefits Annotation
We used two type of data annotation
-
Polygon Annotation
Used for tracking a person’s every small movement.
1. Separation of each movement
From each video each movement was separated based on frame per second to capture the way of response.
2. Preparation and division of batches
The separated frames were prepared and divided into batches on the annotation platform.
3. Labelling
Each body part was labelled by polygon annotation, for e.g. hair, shoulders, torso, head, face.
Processed above 17k images with Quality achievement above client’s benchmark 95.00%
-
Data Segmentation
Used to identify objects in an image at pixel level, it was done in three steps.
1. Image classification
Image classification helped to recognize the objects and existing properties in an image.
2. Object detection
Object detection allowed to move one-step further and find the accurate position of the object of interest, which was done through bounding box annotation.
3. Image segmentation
With image segmentation, we could recognize and understand what exactly is in the image at pixel level view.
Processed above 90k images with Quality achievement above client’s benchmark 95.00%
We prepared a labeling data with metadata in preparation for training a machine learning model for the client. These annotated datasets were then used to train autonomous vehicles, chatbots and translation systems.